Related Blogs
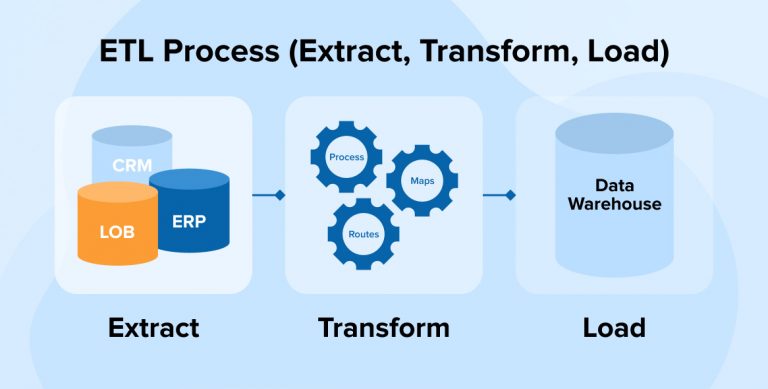
1. Overview
Nowadays by seeing the current market situation, data processing of structured and unstructured data becomes a very crucial part of an effective business. Business partners are investing more in data processing since the amount (volume) and variety of raw data increases very rapidly.
To do effective business, data planning, processing and maintaining are playing very important roles. Since the last decade, the Extract Transform Load process has become fruitful to flow business processes smoothly.
Data mining and Data Warehousing are the key concepts to analyze big data. Also to make the best business decisions and strategies which can increase revenue and profit of an organization.
Data Warehouse is the collection of historical data from multiple sources (homogeneous/heterogeneous data) which is used for data analysis and data querying to retrieve some meaningful and knowledgeable information. Data warehouses allow access to data from multiple systems. In addition, today’s cloud data warehouse and data lake infrastructure provide plenty of storage capacity hence it is no longer required to compress all your data before loading it to the target data warehouse.
2. ETL Process
Data Warehousing is the process to store data from multiple sources to a single data store that is loaded to Data Warehouse which can be a text file, images, spreadsheet, operation data, relational/non-relational data, sensitive data, sensor data, etc., but heterogeneous data may contain ambiguity and redundancy called “Dirty Data“.
To deal with dirty data and increase the data quality some cleaning processing must be applied to that heterogeneous data which is known as “Data Preprocessing“.
After preprocessing raw data, consistent data will be pulled from multiple databases and loaded as a single data store into Data Warehouse or another unified data repository (target system), this entire process is called Extract Transform Load Process“.
The ETL process stands for Extract Transform and Load. ETL processes the streaming data in a very traditional way. It is mainly used for data cleansing, data processing and data loading into the target database. Data analytics and machine learning work streams are built on top of ETL. Here the loaded data is meaningful data that will be used by the end user for different purposes. Below are the main three stages of an ETL Process – a paradigm to process data:
The main characteristic of the Extract Transform Load(ETL) process is that data extraction, data transformation and loading stages to the Data Warehouse can run in parallel.
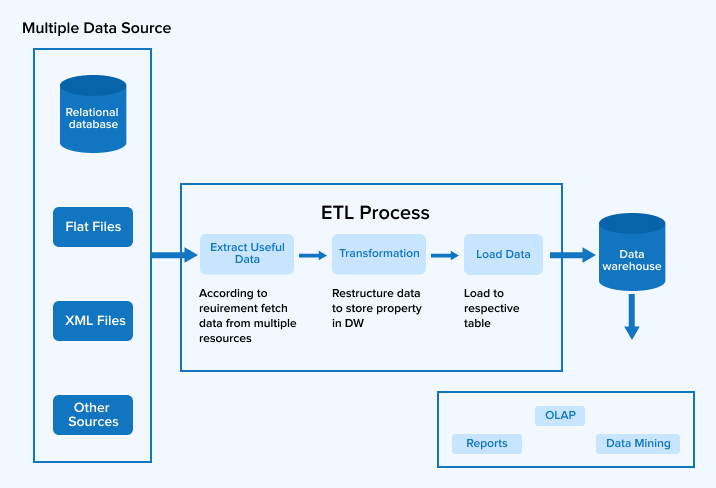
1. Extraction:
Data is captured/extracted from the legacy systems according to the business intelligence requirements with the help of various data analysis tools. The most important step for a successful ETL Process is to extract data and process data from various data sources and Data engineers are responsible to extract data periodically. What to extract depends on the available data sources and business requirements.
To filter the raw data, validation rules are applied in the Extraction process. The extraction process may have to modify the validation rules to accept data for moving to the next phase based on business requirements. It is not a one time process, changes are applied from the source at a regular time period.
There are various methods available to apply changes on extracted data from multiple data sources:
- Notifying System
- Incremental Update
- Full Extraction
- Online Extraction
It is not necessary to store data directly to the traditional data warehouses sometimes according to requirement it is stored into the “Warehouse Staging Area” which is a temporary data storage part of the Data Warehouse. During this stage, we are allowed to generate audit reports for data diagnosis.
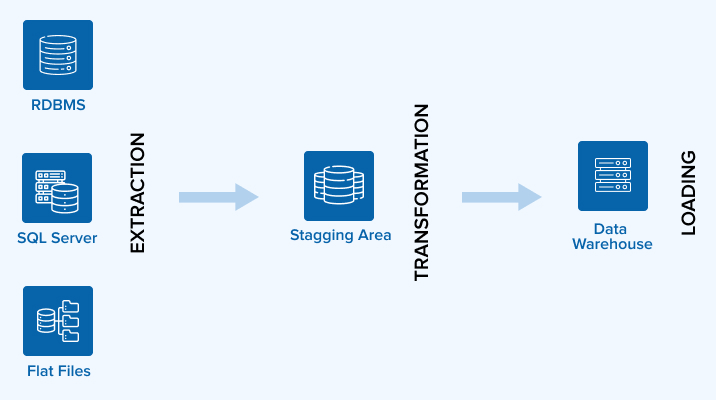
Extracted data are transformed from the data source file to Staging and Staging to Data Warehouse and Warehouse to Data Mart.
2. Transformation:
Extracted Data needs to be transformed into a structured format that is compatible with the predefined Data Warehouse format while ensuring the data quality.
In this phase, a set of rules are applied to transform data into meaningful and structured data which will be used to gain business processes.
It includes following steps that are applied to transform data:
- Cleaning: Data Mapping of particular values by code (i.e. null value to 0, male to ‘m’, female to ‘f’) to ensure data quality.
- Deriving: Generate new values using available values (e.g. Total from price and quantity).
- Sorting: Sort data according to some specific value for fast retrieval.
- Joining: Integrate data elements of multiple sources and generate default values (lookup & merge) while maintaining data quality.
- Generate surrogate key values for uniformity in semantics and performance.
- Validation: During refreshment of DW, some values may be changed which would cause inconsistency in DW. By applying validation rules, we can preserve old values in other fields and replace the original value with new values to handle the “Slowly Change Dimension” problem. There are also more solutions available for this problem nowadays.
After completion of each step, data will be stored into a staging area for further processing.
In case of any failure, the transformation process will be resumed from staging.
3. Load:
Structured/Transformed data will be loaded to its appropriate table in the Data Warehouse.
Data consistency must be maintained because records may be updated at loading time. Also referential integrity should be maintained to preserve data consistency.
The Loading stage could also be used to load data and migrate data to Data Marts (Subject Oriented Data).
Loading of transformed data can be performed in two ways:
- Record by record
- Bulk Load
Bulk load is the most preferable strategy to load data in the warehouse in order to improve performance since it provides less overhead than Record by record strategy.
To improve query performance data tables should be partitioned while loading data to the target system. Table partitioning may depend on time interval strategy (intervals like year, month, quarter etc.).
3. ETL vs ELT
1. What is ETL?
ETL is a contraction of Extract, Transform and Load. It is the process of extracting data from one or more remote sources, performing all the required calculations on it, and storing the sensitive data into the data warehouse system. This process takes place within the target database, which requires only unprepared and raw data.
Basically, it was designed to gather information from relational databases. Now, ETL tools help to implement this process automatically to extract data from several sources and automate the operations.
Process of Extraction
As we can see in the above-mentioned picture, a professionally designed ETL tool extracts data from remote systems and flat files to REST APIs to deliver all application-related information.
Transformation Process
The transformation of data takes place in the staging area after extracting the data. The remote sources may deliver the data various times, so make sure you have a staging area.
Loading process
This is the final level where the ETL tool will collect the mutated data in your warehouse system.
2. What is ELT?
ELT is a tool used for data migration. It extracts data from more than one source system and collects them in a data lake. Thereafter, the data which is extracted from the system is then transformed and loaded into the target destination.
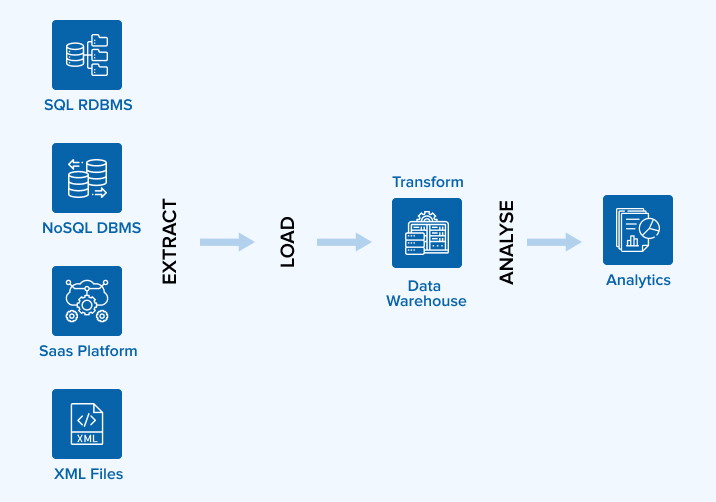
There are two main transformations to take into consideration while you’re migrating from ETL to ELT, that are:
- the utilization of unstructured data increases
- and the popularity of cloud-based storage systems.
Images, audio, and video are the form of raw data which requires a huge amount of time for data integration. As it takes a lot of time to transform, the ETL process may slow down. So, firstly the data is stored in warehouse systems and then the transformations occur as needed.
When migrating to ELT, it is supported by cloud storage technologies. It helps you to gather raw data and work fast and in large-scale data transformations.
4. What are the Benefits of ETL?
- Migrating to ETL helps to improve data quality by cleansing the data before it is loaded to a new repository. For a time-consuming operation, it is recommended to use ETL to create small data repositories that are regularly updated, whereas other migration methods change data capture and data virtualization to integrate high amounts of data.
- Manage data warehouse storage: If your data storage is a cost-sensitive system, you can use ETL to keep the storage costs low as it collects only the required data which reduces the usage of data storage.
- Compliance: Consider some privacy regulations like SOC2 or HIPAA that are specific to your company. Such regulations should be eliminated and encrypted sensitive data before collecting them in your data storehouse. By doing this, you can achieve the ETL process by eliminating data at the transform stage.
5. Key difference between ETL and ELT
- Data is loaded first when using the ETL process whereas ELT loads the information directly into the remote system.
- ELT utilizes the data storehouse to perform basic transformations without using data staging.
- ELT is used for a huge amount of raw or unstructured data whereas the ETL model is used for structured and relational data.
- ETL is used to perform complicated data transformations and it helps to reduce cost.
Have a quick look at the comparison summary of ELT vs ETL:
ETL | ELT | |
Stands for | Extract, Transform, and Load | Extract, Load, and Transform |
Latency | High | Low |
Compliance with security protocols | Easy to implement | Hard to implement, as it requires the data warehouse support |
Storage type | Cloud storage or on-premises | Optimised for cloud data storehouses |
Time maintenance | Requires high maintenance | Requires low maintenance |
Data lake support | No | Yes |
Transformation | Transformations are done in ETL server | Transformations are done in the target system |
6. What are ETL Tools?
As we discussed earlier, ETL allows you to extract data from various resources, transform that data and load all the data into the target data store.
All ETL tools are designed to perform various tasks, but for analytics and data warehousing, it is an important element. These tools are executed to integrate the project that involves three main phases namely extraction, transformation, and loading. It allows you to extract information from one or more resources, do some modifications to make it suitable with the target system, and load it into its target remote system.
The capabilities of such tools includes:
- Automation and easy usability: Using ETL tools allows you to automate process data flow, starting from data sources to the target data destination. Such tools are used for extracting, transforming, and loading data.
- Drag-and-drop feature: The drag-and-drop functionality is used for defining rules and data processes.
- Support complicated data management: It provides support in complex calculating, string manipulations, and data integrations.
- Safety and compliance: Using effective ETL tools allows you to encrypt data in motion and are approved compliant with government regulations such as General data protection regulation and DPR and HIPAA.
Top four things an ETL tool needs to do:
- Cloud-native: Traditional ETL tools are designed for a platform having limitations, as it does not render into traditional data warehouses. These tools will not step up to achieve the benefit of the scalability and speed of the cloud.
- It needs to go deep: ETL is a cloud-based tool, so it is essential to perform all the tasks included in traditional ETL tools. It should be capable enough to take various types of customer data whether it is structured, semi-structured, or on-cloud.
- Transformative: ETL tools should be transformative. Transferring information from one source to another isn’t the whole job. Business organizations are now planning to design a tool that can be used to transform the collected data and get it analytics-ready.
- Flexible: A business mostly has data and operations in more than one cloud. Choosing a reliable tool and using it to transform information from one to another cloud is rarely done. You constantly innovate at the transformation panel, as the industry evolves and new problems require new solutions and insights.
7. Available ETL tools in the market
As part of the data integration process, most firms now use various ETL tools to consolidate data. Let us check out Open Source and Commercial ETL tools.
1. Open Source Tools:
- CloverETL
- GeoKettle
- Jaspersoft ETL
- KETL
- Pentaho’s Data Integration (KETTLE)
- Talend – Talend Open Studio
- IKAN – ETL4ALL
2. Commercial Tools:
- Informatica – Power Center
- IBM – Websphere DataStage(Formerly known as Ascential DataStage)
- SAP – BusinessObjects Data Integrator
- IBM – Cognos Data Manager (Formerly known as Cognos DecisionStream)
- Microsoft – SQL Server Integration Services
- Oracle – Data Integrator (Formerly known as Sunopsis Data Conductor)
- SAS – Data Integration Studio
- Oracle – Warehouse Builder
- AB Initio
- Information Builders – Data Migrator
- Embarcadero Technologies – DT/Studio
- IBM – DB2 Warehouse Edition
- Pervasive – Data Integrator
- ETL Solutions Ltd. – Transformation Manager
- Group 1 Software (Sagent) – DataFlow
- Sybase – Data Integrated Suite ETL
- Expressor Software – Expressor Semantic Data Integration System
- Elixir – Elixir Repertoire
- Business Objects Data Integrator
8. Conclusion
Are you suffering from any kind of data integration constriction? If so, then search for an automated ELT platform that provides a cloud-based and no-code interface that overcomes the data integration challenges and makes it hassle-free.
ETL and ELT both are data integration tools used to transform data and improve data quality for data integration in various ways. Choose the solutions that depend on the data you have, are suitable for storage that you’re using, and are a perfect fit for long-term business requirements.
Subscribe to our Newsletter
Signup for our newsletter and join 2700+ global business executives and technology experts to receive handpicked industry insights and latest news
Build your Team
Want to Hire Skilled Developers?
Comments
Leave a message...